Four Approaches to Face Detection
R87526023 陳必衷
bi@turing.csie.ntu.edu.tw
Download Matlab programs
1. Introduction
目前做Face Detection的方法大致可分為下幾種:Neural Network的方法、Feature-based 的方法及
Color-based的方法。其中,Neural Network的方法是用許多的Training Data(一部份是臉部的、一部份是非臉部的)去訓練Neural Network,使其可以辨識出什麼影像是臉、什麼影像不是臉。Feature-based的方法是利用人臉部的一些特徵來偵測人臉,例如:人臉上有眼睛、鼻子、嘴巴,而這些器官都有固定的相對位置;就整張臉而言,大概是呈橢圓形,而臉和背景之間大致會有邊緣線;諸如此類,有許許多多的特徵可利用。而Color-based的方法,則是利用人臉部的顏色來判斷某影像是否是臉;若影像是彩色的,我們知道臉的顏色是橘黃、黃、白、褐、深褐色;若影像是灰階的,我們知道人臉的灰階顏色的變化並不會太大,而眼睛、嘴巴、頭髮會是較黑的部份。
在這個期末專題中,我用四種方法分別來做人臉偵測,分別是:Neural Network的方法、Rule-based的方法(Feature-based方法的一種)、Elliptical Edge的方法(Feature-based方法的一種)及Average Face的方法(Color-based方法的一種)。假設要偵測的是正面、並且沒有歪斜的臉。在以下各段中,將詳細說明每一個方法的細節、優缺點及可以如何改進。
此專題的目的是測試各方法的優缺點,並不期望增加執行的速度。系統的Input是灰階的影像(包含人臉在其中的影像,人臉皆是正面並且沒有歪斜),Output是原影像並在其中1~5個最像人臉的位置以黑框框出。
2. Framework
因為要找尋不同大小、不同位置的人臉,所以系統要在不同大小、不同位置的區域上來做判斷。在此假設人臉的大小最大為輸入影像的1/2,最小為輸入影像的1/10。所以尋找的方式是先設定『可能人臉方塊(PossibleBlock)』的大小為輸入影像大小的1/2,再來是前一次『可能人臉方塊』大小的0.89倍,直到『可能人臉方塊』的大小小於輸入影像的1/10。(如此尋找區域大概有20種不同的大小)
若『可能人臉方塊』的大小已固定,接下來就是用這個大小的方形,掃過整個輸入影像。Step為『可能人臉方塊』大小的1/10。再來,因各種不同方法的需要,有時我們必須要對『可能人臉方塊』做Down Sample,之後便可判斷此方塊為人臉的可能性。最後便可依據之前計算出來的可能性,列出1~5最有可能為人臉的位置。如此,我們便可以偵測不同大小、不同區域的人臉。
本專題所用的各方法,都是利用這個Framework,所不同的是在判斷『可能人臉方塊』究竟是否為人臉時,有的是用Neural Network、有的是用一些規則、有的是比較灰階的差異。故在以下各段中,僅敘述如何計算『可能人臉方塊』的人臉可能性。
2.1 Framework的詳細步驟
Set BlockSize = ImageSize / 2
If BlockSize < ImageSize / 10 then goto (9) else goto(3)
for x = 1 to ImageWidth step by BlockWidth/10
for y = 1 to ImHeight step by BlockHeight/10
PossibleBlock is the block centered at (x , y) on the input image.
Resize PossibleBlock to proper size
Compute the Possibility that the PossibleBlock is a face region.
Set BlockSize = BlockSize * 0.89 then goto (2)
Select the most possible region as the face detected.
3. Average Face Approach
Average Face 是典型的人臉影像,此方法是在輸入影像中,找尋與這個典型影像最相似的某幾個區域。在此所用的Average Face是16 x 16的影像,所以我們要『可能人臉方塊(PossibleBlock)』縮小成
16 x 16,然後計算此 16 x 16的Block與Average Face的Mean Square Error。並把Error最小的1~5個區域當作人臉部的區域。
3.1 Average Face Approach的詳細步驟
Resize PossibleBlock to 16 x 16
Possibility = Negative of Mean Square Error between Average Face and PossibleBlock.
3.2 Average Face Approach的特性
這是一個非常簡單的方法,對於簡單背景的圖片似乎都還可以找得到臉的部位,但當背景複雜起來時,就會很容易有錯誤。
- 簡單且容易
implement。
可以算出每個『可能人臉方塊』與人臉的相似度數值。
- 背景複雜時很容易錯誤。
- 人臉的亮度太黑或太亮時都很容易出錯。
- 人臉表情太大時也易出錯。
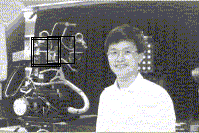
偵測到覆雜的區域而非人臉
當背景簡單時可偵測到人臉
用途:
- 背景為較單純的黑色或白色時可以很快找到人臉。
- 因為此方法可以算出每個『可能人臉方塊』與人臉的相似度數值,所以可以結合於一些用Rule或Neural Network﹍﹍等不易計算相似度數值的方法。在這樣的結合中,Rule或Neural Network扮演filter的角色,把不像人臉的區域過濾掉,最後再用Average Face取出最像臉的1~5個區域。
4. Rule-based Approach
Rule-based方法是用一些人臉上的一些規則來判斷人臉的存在,如:在灰階的影像中,眼睛的部位較暗,並且兩個眼睛會成對出現。當然,我們只要仔細的觀察,便可以列出許許多多的規則,但並不是每個都適用。
在這裡我所提出的方法是先用4 x 4的Mosaic Image來大概判斷是否為人臉,若像人臉的可能性蠻高的,則再用
8 x 8的Mosaic Image來找出眼睛的位置【Ref 1】。當然若找不出眼睛,這塊區域就應該不會是人臉了。之後再用Average Face的方法計算此區域為人臉的可能性。最後列出最可能的1~5人臉區域。
4.1 Rule-based Approach 詳細步驟
Resize PossibleBlock to 4 x 4
Apply 4 x 4 Rules:
- The center part of the face has 4 cells with a basically uniform gray level. (mean of difference is less then 30)
- The upper round part of face has a basically uniform grey level. (mean of difference is less then 70)
- The difference between the average gray levels of 1, 2.is significant. (the difference is large then 50)
- If the PossibleBlock obeys the 4 x 4 Rules then goto (4) else Possibility = 0 and STOP.
- Apply 8 x 8 Rules to find EYES:
- There is a local minimum of gray level along vertical direction.
- In the horizontal direction, there are two local minimum of gray level, the distance between these minimum d: 2 < d < 5. The 2 local minimum are called Eye Pairs.
- Number of Eye Pairs in one 8 x 8 block is less then 7.
- If tht PossibleBlock obeys the 8 x 8 Rules then goto (6) else Possibility = 0 and STOP
- Use the Eye Pairs found in step (4) to reform the PossibleBlock. Ensure that eye positions are at the right positions.
- Use the reformed PossibleBlock to compute Mean Square Error between this block and average face. And the Possibility = the Mean Square Error.
4.2 Rule-based Approach的特性
Rule-based的方法是利用人臉部灰階的一些規則來判斷人臉的存在,再
4x4的Rule中,我們假設人臉部的顏色會比臉上面的顏色亮一些,這個規則對於有頭髮並膚色較淡的人臉而言是有效的,但若輸入影像中的人是光頭或是黑人,則此方法遍佈適用了。再者在8 x 8的Rule中,我們主要是希望能找到Eye Pairs,並利用此Eye Pairs來校正PossibleBlock的位置。在此我們假設人眼睛的部位會有一個灰階的極小值,這個假設是很合理的,並且在一些paper中也指出,人臉上的眼睛是比較不會隨人表情變化的特徵。因此只要輸入影像的人不是光頭或黑人,這個方法應該大致適用。
- 判斷出來的位置幾乎就一定是人臉。。
- 用階層(Hierarchical)的搜尋法,會使執行速度增加。
- 4 x 4 Rule無法判定出光頭及膚色很黑(或亮度很暗)的人臉。
- 4 x 4 Rule有可能會找不道人臉,即使輸入影像中有人臉。
(1) 此方法適用於頭髮深色、臉部較明亮的人臉。
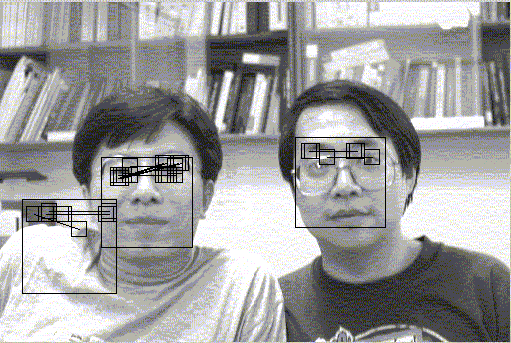
實際抓到的人臉,可能為眼睛的區域也由方框框出。
5. Elliptical Edge Approach
Elliptical Edge的方法是假設人臉都是近似橢圓的形狀,並且假設人臉與背景之間有一條較明顯的邊緣線。所以我們只要在輸入影像中尋找有橢圓邊緣的區域,在用此區域和Average Face做比較,最後便可框出最像人臉的1~5個區域。在判斷橢圓邊緣時,我用有橢圓邊緣與輸入影像的邊緣影像做convolution,並取出最值最大點作為PossibleBlock的中心點。
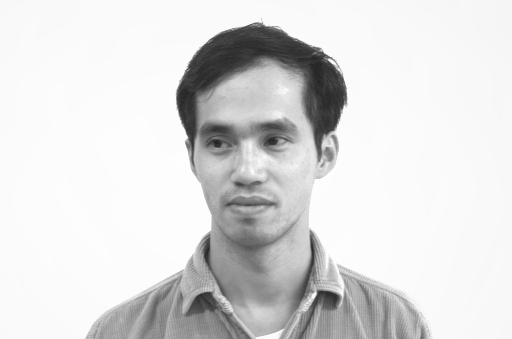


5.1 Elliptical Edge Approach的詳細步驟
此方法與其他三個方法比較不同,並不適用第二節中所提出來的Framework,此方法也是將PossibleBlock由輸入影像的1/2找到1/10。只是此方法不用將PossibleBlock掃過整張輸入影像並且一一比對,而是用convolution的方法,一次就定位出最可能的區域。用convolution的好處是他可以使用FFT,如此便可增加執行速度。
- Find edges of the input image using Sobel operator.
- Convolute the edge image with elliptical edge image.
- Find 5~10 points which have the maximum value.
- Form the PossibleBlocks centered at the points found in step (3).
- Compute the Mean Square Error between the PossibleBlocks and the average face. And set Possibility = the Mean Square Error.
5.2 Elliptical Edge Approach的特性
Elliptical Edge Approach是個執行速度很快的方法,但誤判的機會也很高。只要人臉與其背景並沒有明顯的邊緣線,這個方法基本上就失效了。或者當背景中有明顯的一塊白色區域或黑色區域,這個方法就會誤判其為人臉。
- 簡單快速。
- 當人臉區域明顯時,效果不錯。
- 當人臉與背景沒有明顯邊緣時便找不到人臉。
- 當背景中有明顯邊緣時,可能會誤判此為人臉。
- 當背景有複雜邊緣線時,也容易誤判為人臉。
(1) 當背景較為單純時,此方法是快速有效的方法。
- 此方法最大的缺點是較容易找不到人臉,所以應該要讓PossibleBlock的數量增加,並且在判斷Edge時做Normalization。如此會有較多的區域進行average face的比對,如此應該可以較容易找到人臉。
- 可以用Image processing中Enhance Edge的方法對輸入影像做前處理,如此人臉的邊緣應該會較為明顯。
- 可用一些Morphological operation的方法把細小的邊緣清除,如此可減少False Detection的區域。
6. Neural Network-based Approach
Neural Network的方法是用許多人臉及非人臉的影像去訓練Neural Network,使其可以學到什麼是人臉,什麼不是人臉。在偵測時就直接把PossibleBlock的每一個pixel喂到Neural Network中,如果此區塊為人臉則output 1,否則output 0。
Neural Network的架構用Feed-forward 的Back-propagation Neural Network,Input layer是20 x 23的image,Output layer只有一個Neuron,若是人臉output為1,否則為0。Hidden layer只有一層,總共20個Neuron。而Transfer Function,在Hidden layer中是用從-1到1的sigmoid function;在Output layer中則是用從0到1的sigmoid function。Train Set包含1000張人臉影像、4000張非人臉影像。
6.1 Neural Network-based Approach的詳細步驟
Network Properties:
3 layer ( 1 hidden layer ) backpropagation network.
Input layer: 460 nodes, and range from 0 to 255 ( gray level )
Output layer: 1 nodes, and range form 0 to 1 ( face:1, non-face: 0 )
Hidden layer: 20 nodes.
Transfer function:
Hidden layer: Sigmoid function, range from -1 to 1.
Output layer: Sigmoid function, range form 0 to 1.
- Train Set: 1000 positive examples, 4000 negative examples
Training Procedure:
- Initial network. Randomly assign all weights.
- Initial training set. Normalize training set or do PCA.
- Batch training (use Resilient backpropagation) until:
Mean Square Error < 10 -5 or
Epoch > 400 or
Training time > 1 hour
- Set Threshold = 1/2 + ( min face output + max non-face output ) / 4
- Use the Threshold to test the network performance of Test Set. (Test Set consist of 100 faces and 500 non-faces)
- If there are still unused Non-face Set (total number of Non-face blocks is 8000) , put the Non-face Set to Training Set, and goto (2) else goto (7)
- Test network performance. If there is NO error of Test Set, then STOP else goto (8).
- If the network has best performance then store the network.
- Goto (1)
6.2 Neural Network-based Approach的特性
Neural Network方法的好壞,與Training Data的好壞有密切的關係,因為我所用的Training Data都是自己由一張張包含人臉的影像中剪下來的,所以Training Data的大小沒有辦法太大,因此偵測人臉的效果也因此不太好。而Neural有與多的參數可以調整,例如:Hidden layer的個數及層數、transfer function的類型、training的方法……等等,所以要調到最佳的狀況是一件很不容易的事。我想我的network應該並沒有到最佳狀況,所以大概會有2 %的false detection。而人臉的位置通常一定可以偵測到。
- 通常人臉的位置一定可以偵測到。
- 訓練Neural Network很自動化的動作,所以可以讓電腦自己跑。
- 偵測的好壞與Training Set的好壞非常相關。
- Network的參數很難調整到最佳狀況。
- 在我的實驗中,False detection的比率稍高。
- 可以達到偵測效果的原因較難明瞭,因此也較難做微調。
- 執行速度是四種中最慢的。
- 改進Training Set。
- 可用cross-correlation operation增快執行速度【Ref 4】。
7. Conclusion
經過一些實驗,發現Average face approach適合做人臉偵測的最後確認;Rule-based approach適合偵測深色頭髮、膚色較淺的人臉,而且判斷的正確率算是相當的高;Elliptical edge approach適用於臉部輪廓明顯的影像,而且速度相當快;而Neural Network則可適用於一般的人臉偵測,但是好的Training data不一取得,而Network的參數又不易調整。
其實一般來說,要讓Face detection做得又快有準,輸入影像通常要先做前置處理,例如:Morphological operations 【Ref 3】…等。並且可以整合多種方法加以判斷,然此精準對便可提升。在此這個期末專題中,我嘗試了4種Face detection的方法,並整理出其優缺點,相信對於日後的方法整合可以提供一些建議。
Reference
Guangzheng Yang and Thomas S. Huang, "Human Face Detection in a Complex Background", Pattern Recognition, Vol. 27. No. 1 ,1994.
Kazuhiro Hotta , Takio Kurita and Taketoshi Moshima, "Scale Invariant Face Detection Method using Higher-Order Local Autocorrelation Features extracted from Log-Polar Image", IEEE 1998
Chin-Chung Han, Hong-Yuan Mark Liao, Kuo-Chung Yu, and Liang-Hua Chen, "Fast Face Detection via Morphology-based Pre-processing", Academia Sinica, Taipei, Taiwan.
Beat Fasel, Souleil Ben-Yacoub, Juergen Luettin and Stphane Marchand-Maillet, "Fast Multi-Scale Face Detection", IDIAP-Com 98-04