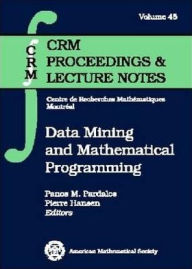
Data Mining and Mathematical Programming
by Panos M. Pardalos, P. Hansen
Data mining aims at finding interesting, useful or profitable information in very large databases. The enormous increase in the size of available scientific and commercial databases (data avalanche) as well as the continuing and exponential growth in performance of present day computers make data mining a very active field. In many cases, the burgeoning volume of
… See more details belowOverview
Data mining aims at finding interesting, useful or profitable information in very large databases. The enormous increase in the size of available scientific and commercial databases (data avalanche) as well as the continuing and exponential growth in performance of present day computers make data mining a very active field. In many cases, the burgeoning volume of data sets has grown so large that it threatens to overwhelm rather than enlighten scientists. Therefore, traditional methods are revised and streamlined, complemented by many new methods to address challenging new problems. Mathematical Programming plays a key role in this endeavor. It helps us to formulate precise objectives (e.g., a clustering criterion or a measure of discrimination) as well as the constraints imposed on the solution (e.g., find a partition, a covering or a hierarchy in clustering). It also provides powerful mathematical tools to build highly performing exact or approximate algorithms. This book is based on lectures presented at the workshop on Data Mining and Mathematical Programming" (October 10-13
Product Details
- ISBN-13:
- 9780821843529
- Publisher:
- American Mathematical Society
- Publication date:
- 04/09/2008
- Series:
- CRM Proceedings and Lecture Notes Series, #45
- Pages:
- 234
- Product dimensions:
- 6.90(w) x 9.90(h) x 0.50(d)
Table of Contents
Support vector machines and distance minimization Emilio Carrizosa Carrizosa, Emilio 1
0-1 semidefinite programming for graph-cut clustering : modelling and approximation Huarong Chen Chen, Huarong Jiming Peng Peng, Jiming 15
Artificial attributes in analyzing biomedical databases Zsolt Csizmadia Csizmadia, Zsolt Peter L. Hammer Hammer, Peter L. Bela Vizvari Vizvari, Bela 41
Recent advances in mathematical programming for classification and cluster analysis Ya-Ju Fan Fan, Ya-Ju Cem Iyigun Iyigun, Cem W. Art Chaovalitwongse Chaovalitwongse, W. Art 67
Nonlinear skeletons of data sets and applications - methods based on subspace clustering Pando G. Georgiev Georgiev, Pando G. 95
Current classification algorithms for biomedical applications Mario R. Guarracino Guarracino, Mario R. Salvatore Cuciniello Cuciniello, Salvatore Davide Feminiano Feminiano, Davide Gerardo Toraldo Toraldo, Gerardo Panos M. Pardalos Pardalos, Panos M. 109
Bilevel model selection for support vector machines Gautam Kunapuli Kunapuli, Gautam Kristin P. Bennett Bennett, Kristin P. Jing Hu Jing, Hu Jong-Shi Pang Pang, Jong-Shi 129
Algorithms for detecting complete and partial horizontal gene transfers : theory and practice Vladimir Makarenkov Makarenkov, Vladimir Alix Boc Boc, Alix Alpha Boubacar Diallo Diallo, Alpha Boubacar Abdoulaye Banire Diallo Diallo, Abdoulaye Banire 159
Nonlinear knowledge in kernel machines Olvi L. Mangasarian Mangasarian, Olvi L. Edward W. Wild Wild, Edward W. 181
Ultrametric embedding : application to data fingerprinting and to fast data clustering Fionn Murtagh Murtagh, Fionn 199
Selective linear and nonlinear classification Onur Seref Seref, Onur O. ErhunKundakcioglu Kundakcioglu, O. Erhun Panos M. Pardalos Pardalos, Panos M. 211
Customer Reviews
Average Review: