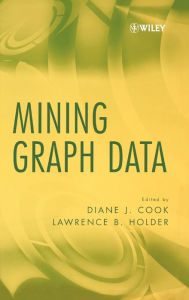
Mining Graph Data / Edition 1
by Diane J. Cook
Discover the latest data mining techniques for analyzing graph data
This text takes a focused and comprehensive look at an area of data mining that is quickly rising to the forefront of the field: mining data that is represented as a graph. Each chapter is written by a leading researcher in the field; collectively, the chapters represent the latest
/b>… See more details belowOverview
Discover the latest data mining techniques for analyzing graph data
This text takes a focused and comprehensive look at an area of data mining that is quickly rising to the forefront of the field: mining data that is represented as a graph. Each chapter is written by a leading researcher in the field; collectively, the chapters represent the latest findings and applications in both theory and practice, including solutions to many of the algorithmic challenges that arise in mining graph data. Following the authors' step-by-step guidance, even readers with minimal background in analyzing graph data will be able to represent data as graphs, extract patterns and concepts from the data, and apply the methodologies presented in the text to real datasets.
Mining Graph Data is divided into three parts:
- Part I, Graphs, offers an introduction to basic graph terminology and techniques.
- Part II, Mining Techniques, features a detailed examination of computational techniques for extracting patterns from graph data. These techniques are the state of the art in frequent substructure mining, link analysis, graph kernels, and graph grammars.
- Part III, Applications, describes the application of data mining techniques to four graph-based application domains: chemical graphs, bioinformatics data, Web graphs, and social networks.
Practical case studies are included in many of the chapters. An accompanying Web site features source code and datasets, offering readers the opportunity to experiment with the techniques presented in the book as well as test their own ideas on graph data. The Web site also includes the results of many of the techniques presented in the text.
This landmark work is intended for students and researchers in computer science, information systems, and data mining who want to learn how to analyze and extract useful patterns and concepts from graph data.
Product Details
- ISBN-13:
- 9780471731900
- Publisher:
- Wiley
- Publication date:
- 11/28/2006
- Edition description:
- New Edition
- Pages:
- 500
- Product dimensions:
- 6.44(w) x 9.23(h) x 1.31(d)
Table of Contents
Preface.
Acknowledgments.
Contributors.
1 INTRODUCTION (Lawrence B. Holder and Diane J. Cook).
1.1 Terminology.
1.2 Graph Databases.
1.3 Book Overview.
References.
Part I GRAPHS.
2 GRAPH MATCHING—EXACT AND ERROR-TOLERANT METHODS AND THE AUTOMATIC LEARNING OF EDIT COSTS (Horst Bunke and Michel Neuhaus).
2.1 Introduction.
2.2 Definitions and Graph Matching Methods.
2.3 Learning Edit Costs.
2.4 Experimental Evaluation.
2.5 Discussion and Conclusions.
References.
3 GRAPH VISUALIZATION AND DATA MINING (Walter Didimo and Giuseppe Liotta).
3.1 Introduction.
3.2 Graph Drawing Techniques.
3.3 Examples of Visualization Systems.
3.4 Conclusions.
References.
4 GRAPH PATTERNS AND THE R-MAT GENERATOR (Deepayan Chakrabarti and Christos Faloutsos).
4.1 Introduction.
4.2 Background and Related Work.
4.3 NetMine and R-MAT.
4.4 Experiments.
4.5 Conclusions.
References.
Part II MINING TECHNIQUES.
5 DISCOVERY OF FREQUENT SUBSTRUCTURES (Xifeng Yan and Jiawei Han).
5.1 Introduction.
5.2 Preliminary Concepts.
5.3 Apriori-based Approach.
5.4 Pattern Growth Approach.
5.5 Variant Substructure Patterns.
5.6 Experiments and Performance Study.
5.7 Conclusions.
References.
6 FINDING TOPOLOGICAL FREQUENT PATTERNS FROM GRAPH DATASETS (Michihiro Kuramochi and George Karypis).
6.1 Introduction.
6.2 Background Definitions and Notation.
6.3 Frequent Pattern Discovery from Graph Datasets—Problem Definitions.
6.4 FSG for the Graph-Transaction Setting.
6.5 SIGRAM for the Single-Graph Setting.
6.6 GREW—Scalable Frequent Subgraph Discovery Algorithm.
6.7 Related Research.
6.8 Conclusions.
References.
7 UNSUPERVISED AND SUPERVISED PATTERN LEARNING IN GRAPH DATA (Diane J. Cook, Lawrence B. Holder, and Nikhil Ketkar).
7.1 Introduction.
7.2 Mining Graph Data Using Subdue.
7.3 Comparison to Other Graph-Based Mining Algorithms.
7.4 Comparison to Frequent Substructure Mining Approaches.
7.5 Comparison to ILP Approaches.
7.6 Conclusions.
References.
8 GRAPH GRAMMAR LEARNING (Istvan Jonyer).
8.1 Introduction.
8.2 Related Work.
8.3 Graph Grammar Learning.
8.4 Empirical Evaluation.
8.5 Conclusion.
References.
9 CONSTRUCTING DECISION TREE BASED ON CHUNKINGLESS GRAPH-BASED INDUCTION (Kouzou Ohara, Phu Chien Nguyen, Akira Mogi, Hiroshi Motoda, and Takashi Washio).
9.1 Introduction.
9.2 Graph-Based Induction Revisited.
9.3 Problem Caused by Chunking in B-GBI.
9.4 Chunkingless Graph-Based Induction (Cl-GBI).
9.5 Decision Tree Chunkingless Graph-Based Induction (DT-ClGBI).
9.6 Conclusions.
References.
10 SOME LINKS BETWEEN FORMAL CONCEPT ANALYSIS AND GRAPH MINING (Michel Liquière).
10.1 Presentation.
10.2 Basic Concepts and Notation.
10.3 Formal Concept Analysis.
10.4 Extension Lattice and Description Lattice Give Concept Lattice.
10.5 Graph Description and Galois Lattice.
10.6 Graph Mining and Formal Propositionalization.
10.7 Conclusion.
References.
11 KERNEL METHODS FOR GRAPHS (Thomas Gärtner, Tamás Horváth, Quoc V. Le, Alex J. Smola, and Stefan Wrobel).
11.1 Introduction.
11.2 Graph Classification.
11.3 Vertex Classification.
11.4 Conclusions and Future Work.
References.
12 KERNELS AS LINK ANALYSIS MEASURES (Masashi Shimbo and Takahiko Ito).
12.1 Introduction.
12.2 Preliminaries.
12.3 Kernel-based Unified Framework for Importance and Relatedness.
12.4 Laplacian Kernels as a Relatedness Measure.
12.5 Practical Issues.
12.6 Related Work.
12.7 Evaluation with Bibliographic Citation Data.
12.8 Summary.
References.
13 ENTITY RESOLUTION IN GRAPHS (Indrajit Bhattacharya and Lise Getoor).
13.1 Introduction.
13.2 Related Work.
13.3 Motivating Example for Graph-Based Entity Resolution.
13.4 Graph-Based Entity Resolution: Problem Formulation.
13.5 Similarity Measures for Entity Resolution.
13.6 Graph-Based Clustering for Entity Resolution.
13.7 Experimental Evaluation.
13.8 Conclusion.
References.
Part III APPLICATIONS.
14 MINING FROM CHEMICAL GRAPHS (Takashi Okada).
14.1 Introduction and Representation of Molecules.
14.2 Issues for Mining.
14.3 CASE: A Prototype Mining System in Chemistry.
14.4 Quantitative Estimation Using Graph Mining.
14.5 Extension of Linear Fragments to Graphs.
14.6 Combination of Conditions.
14.7 Concluding Remarks.
References.
15 UNIFIED APPROACH TO ROOTED TREE MINING: ALGORITHMS AND APPLICATIONS (Mohammed Zaki).
15.1 Introduction.
15.2 Preliminaries.
15.3 Related Work.
15.4 Generating Candidate Subtrees.
15.5 Frequency Computation.
15.6 Counting Distinct Occurrences.
15.7 The SLEUTH Algorithm.
15.8 Experimental Results.
15.9 Tree Mining Applications in Bioinformatics.
15.10 Conclusions.
References.
16 DENSE SUBGRAPH EXTRACTION (Andrew Tomkins and Ravi Kumar).
16.1 Introduction.
16.2 Related Work.
16.3 Finding the densest subgraph.
16.4 Trawling.
16.5 Graph Shingling.
16.6 Connection Subgraphs.
16.7 Conclusions.
References.
17 SOCIAL NETWORK ANALYSIS (Sherry E. Marcus, Melanie Moy, and Thayne Coffman).
17.1 Introduction.
17.2 Social Network Analysis.
17.3 Group Detection.
17.4 Terrorist Modus Operandi Detection System.
17.5 Computational Experiments.
17.6 Conclusion.
References.
Index.
Customer Reviews
Average Review: