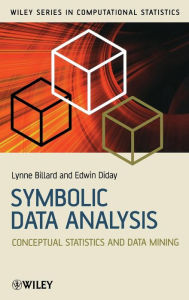
Symbolic Data Analysis: Conceptual Statistics and Data Mining / Edition 1
by Lynne Billard, Edwin Diday
With the advent of computers, very large datasets have become routine. Standard statistical methods don’t have the power or flexibility to analyse these efficiently, and extract the required knowledge. An alternative approach is to summarize a large dataset in such a way that the resulting summary dataset is of a manageable size and yet retains as much of the
… See more details belowOverview
With the advent of computers, very large datasets have become routine. Standard statistical methods don’t have the power or flexibility to analyse these efficiently, and extract the required knowledge. An alternative approach is to summarize a large dataset in such a way that the resulting summary dataset is of a manageable size and yet retains as much of the knowledge in the original dataset as possible. One consequence of this is that the data may no longer be formatted as single values, but be represented by lists, intervals, distributions, etc. The summarized data have their own internal structure, which must be taken into account in any analysis.
This text presents a unified account of symbolic data, how they arise, and how they are structured. The reader is introduced to symbolic analytic methods described in the consistent statistical framework required to carry out such a summary and subsequent analysis.
- Presents a detailed overview of the methods and applications of symbolic data analysis.
- Includes numerous real examples, taken from a variety of application areas, ranging from health and social sciences, to economics and computing.
- Features exercises at the end of each chapter, enabling the reader to develop their understanding of the theory.
- Provides a supplementary website featuring links to download the SODAS software developed exclusively for symbolic data analysis, data sets, and further material.
Primarily aimed at statisticians and data analysts, Symbolic Data Analysis is also ideal for scientists working on problems involving large volumes of data from a range of disciplines, including computer science, health and the social sciences. There is also much of use to graduate students of statistical data analysis courses.
Product Details
- ISBN-13:
- 9780470090169
- Publisher:
- Wiley
- Publication date:
- 02/02/2007
- Series:
- Wiley Series in Computational Statistics Series, #636
- Edition description:
- New Edition
- Pages:
- 330
- Product dimensions:
- 6.30(w) x 9.02(h) x 0.94(d)
Table of Contents
1. Introduction.
References.
2. Symbolic Data.
2.1 Symbolic and Classical Data.
2.2 Categories, Concepts and Symbolic Objects.
2.3 Comparison of Symbolic and Classical Analysis.
3. Basic Descriptive Statistics: One Variate.
3.1 Some Preliminaries.
3.2 Multi-valued Variables.
3.3 Interval-valued Variables.
3.4 Multi-valued Modal variables.
3.5 Interval-valued Modal Variables.
4. Descriptive Statistics: Two or More Variates.
4.1 Multi-valued Variables.
4.2 Interval-valued Variables.
4.3 Modal Multi-valued Variables.
4.4 Modal Interval-valued Variables.
4.5 Baseball Interval-valued Dataset.
4.6 Measures of Dependence.
5. Principal Component Analysis.
5.1 Vertices Method.
5.2 Centers Method.
5.3 Comparison of the Methods.
6. Regression Analysis.
6.1 Classical Multiple Regression Model.
6.2 Multi-valued Variables.
6.3 Interval-valued Variables.
6.4 Histogram-valued Variables.
6.5 Taxonomy Variables.
6.6 Hierarchical Variables.
7. Cluster Analysis.
7.1 Dissimilarity and Distance Measures.
7.2 Clustering Structures.
7.3 Partitions.
7.4 Hierarchy-Divisive Clustering.
7.5 Hierarchy-Pyramid Clusters.
Data Index.
Author Index.
Subject Index.
Customer Reviews
Average Review: